Technology development of deep tech is difficult. Many of such technologies take many years to develop and double or triple time to perfect. If we can identify the common challenges involved in product development process, we can better anticipate and address the issues. (Part B)
In my previous post, I explored three of the unique technological challenges that deep tech faces during product development. In this article, I share how technological complexity of deep tech affects product strategy through three uncertainties characteristic of deep tech products.
Breaking down the uncertainties
4. Peak performance uncertainties
For any deep tech product that is revolutionary, the first product is expected to have terrible performance. Investors, innovators and early adopters alike are sympathetic to this fact. They are willing to pour money into the promise of the product instead of the current state of the product. An important question that they will commonly ask, however, is: What is the best performance the product can deliver if developed to “realistic perfection”? The answer to the question helps investors and customers gauge their prospective return of investment. For instance, considering the burgeoning ethical, environmental and sustainability concerns around food supply, I might be interested in investing in companies developing synthetic food products. However, the cost of production is high and scale of production is low. As an astute investor, I would like to know how much the cost can be reduced and how much the capacity can be increased with reasonable product development efforts. However, I would also be disappointed to find out that answers to the questions are little more than wild guesses where deep tech is concerned. When the rubber hits the road, there will be severe delays at achieving the said performance target. This is because the technology is new and developing, the acme is yet unknown and thus reliable forecasts are challenging.

How product performance improves with rounds after rounds of product development cycles
The figure above is a good representation of how product performance evolves with product development cycles. This model is true right after modules integration and key feasibility studies (refer to previous article Deep Tech Product development with minimal waste) as the product development team would have their first glimpse of how the assembled prototype performs. That performance is denoted as “X”. In deep tech, I have never seen a first prototype that is immediately ready for product launch so chances are, at least 1 additional round of product development is necessary. Since subsequent product development cycles are driven by areas of underperforming metrics from the previous cycle, the goal of development and issues targeted are more focused if correctly managed. The expected marginal performance improvements of a single product development cycle are generally more predictable. With that, performance is improved to point “Y”. However, “Y” may or may not be “Z”, which is the peak performance of the technology if developed to reasonable perfection. The amount of additional performance gains possible with an unlimited number of product development cycles is shrouded in mystery for innovative deep tech products.
The value of “Z” is also highly relevant to the product development team because this information directly feeds into both short-term and long-term business value calculations. The better the performance of the product, the greater value the product commands. In addition, during allocation of development resources, products that can potentially achieve higher performance should be prioritized over products that cannot provide anymore performance improvements. For example, if we consider the efficiency rating of cars, the fuel efficiency of gas-powered vehicles is probably already close to its peak while the efficiency of electric vehicles (in miles per kWh) still has significant room for improvements. Thus, better clarity on the peak performance of the product helps teams focus on products with greater growth and untapped potentials.
It is necessary to acknowledge that peak performance uncertainties pose no challenge to the development of the technology itself. Rather, it affects value creation, strategic/tactical decisions and internal/external communication management. As such, the core principle of dealing with such issues is to build the product strategy, roadmap and process around these uncertainties.
Peak performance uncertainties centered product strategy starts at the product conceptualisation phase. Here, create a comprehensive list of potential applications of the product and their respective performance requirements. Using the example of EV, applications might be city cars, cross-country use, SUVs, all-wheel drives etc. Once this is done, pick the application with the lowest performance requirements and set this as the tentative target application before executing application driven prototype development. Completed prototypes will provide information about the value of “X”. At this point, the team will have a better idea of the performance delta between desired value and “X” along with greater clarity about the technology. Convene a high level meeting to discuss potential product strategies and roadmap revisions. A re-alignment meeting in the middle of product development can help the product generate more value as one of the two situation can occur:
- The team is confident that an additional round of product development will achieve performance value “Y”, which can satisfy multiple different applications. Thus, instead of sticking with the tentative product application, pivot over to the application with greatest anticipated value generation.
- The prototype severely underperformed. Look into other market opportunities to bridge the performance gap. Examples might be looking into applications with even lower performance requirements, understanding the possibilities of partial application offerings, using partnerships to fix the underperforming segment of the technology. In the worst-case scenario, the product might be killed should the outlook be doom and gloom. On the bright side, valuable resources are conserved.
However, what exactly is the value of “Z”? If the financial situation allows, companies can also reap long-term benefits by allocating a small team to explore the limits of the technology. I like to call this team of scientists and engineers the vanguard. Works performed by vanguard lie squarely in the realm of R&D with unclear value proposition and is a long way from productization. Only allocate excess funds to it and pick passionate, independent thinkers to explore the frontiers as it can be an endless, lonely journey. This initiative should run in parallel with the main product development process but both teams must be in frequent communication to exchange information to avoid repeated work or going down the wrong rabbit hole. For the vanguard, I favour the list->check->eliminate R&D process. Scientists and engineers should create a list of research items that they believe are necessary for performance improvement. In the “check” step, run the necessary experiments to prove or disprove the point. If performance indeed improves, yay but if not, it is alright too. Either way, eliminate the item off the list and share the findings with the main product development team. Updating the list is very important, as qualitative projections can be made off the list. For instance, if there are 20 items on the list, 10 of which were tested and performance improvements were negligible thus far, management should assume that the current performance is close to peak performance.
While it is common practice to channel bulk of the resources to the vanguard team, it is generally a mistake especially for smaller companies with shorter runways. Perfecting performance metrics of technology is a necessary component of a product, but it is not the entirety of a product. Even upon fulfilling the performance requirements, multiple miscellaneous steps are still required. If the focus is not on the product, over-development and under-development of various performance metrics generally ensue. This can result in massive delays to product launch.
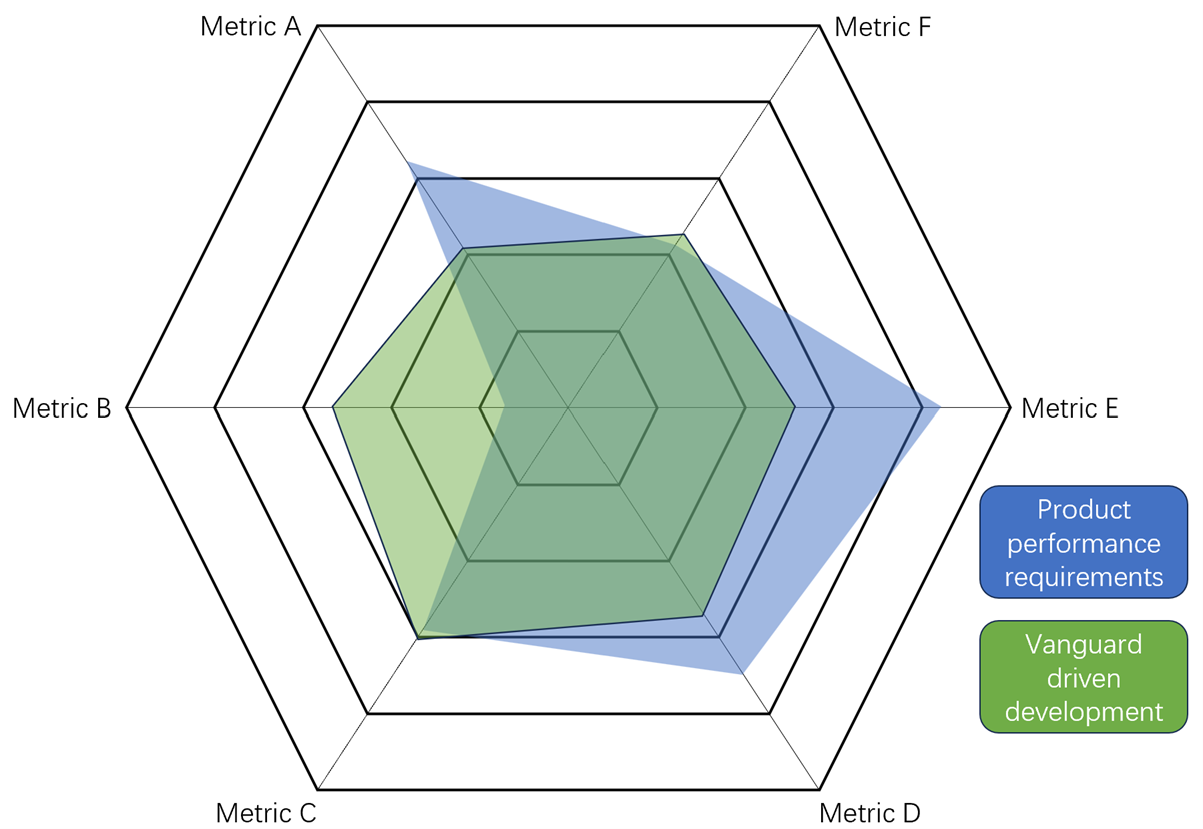
Unfocused development using vanguard-centric approach
In this figure, a vanguard driven development framework results in performance metrics represented by the green area. However, for the target application to work, performance requirements should match the blue area. The area covered by green but not covered by blue represents wasted effort which translates to delays.
5. Metric uncertainties
Much of DNA sequencing currently is done through NGS. Sequencing accuracy, mapped reads, volume of data generated, quality scores etc. are metrics that are widely used to measure the performance of sequencing data. These seemingly commonsense metrics did not appear in a vacuum. How did they start? How were they adopted? Are there alternative metrics that could be adopted instead? Product development teams working on novel deep tech products should ponder upon these questions deeply because chances are they will face metrics adoption uncertainties for the very product they are developing. Metrics allow external customers to be more technically conversant with the product and to draw their own comparative judgements between products. Metrics also allow internal product development team to track their progress and set proper product development goals. Looking at the right set of metrics is more important than hitting the right numbers for irrelevant metrics.
Novel deep tech products are characterized by the fact that they are very different from the next most similar product in the market. For instance, when NGS first came about, they are significantly different from sanger sequencing methods. In most cases, these products will require their own set of metrics to measure performance. Unfortunately, they can get limited inspiration from established products in the market and so many metrics have to be created from scratch or tweaked from established metrics. In this process, the what and the how are both equally important.
- What metrics to use for performance measurements?
- How should these metrics be computed?
To develop product efficiently despite metrics uncertainties, focus first on building a robust metrics governance process.
- Determine the metrics early: Evaluation metrics should be set during the conceptualization phase of product development. Any project manager will agree that a good project should have defined scope and requirements that are decided during the planning phase of project management. The same principle holds here. Clearly outline all the metrics requirements and the respective measurement methods before developing the product.
- Maintain document to track metric evolution: Metrics changes during product development. It is common and inevitable for deep tech products as new discoveries enable better evaluation mechanisms. For instance, I am very sure DNA sequencing quality score is a metric that was introduced mid-way through the product development phase. The method of quality score computation probably went through multiple iterations too during product development. Knowing that changes are unavoidable means we should have a system to track the changes. Tracking metrics evolution can be as easy as having an excel with 6 columns: Metrics name, measurement method, start date, end date, justification, reason for removal. Note that when measurement methods are updated, reported product performances are also affected so this document can also help with performance issue troubleshooting.
- Quality report: Upon being fully developed and ready for market launch, most products would be accompanied by a quality report. The most important technical details of the report are the metrics. I recommend creating mock quality reports as early as possible. Create quality reports for all the intermediate prototypes. This practice allows for a smoother transition to the testing and launch phase of product development and helps to raise hidden issues with the metrics being use. For example, if some of the metrics are qualitative in nature and evaluated through eyeballing, proper training and standardization are crucial for results reliability. Some metrics can only be measured by external vendors which might be time-consuming. In such cases, developing alternatives or more efficient workflow might be necessary.
- Frequent review: For deep tech products, metrics are not cast to stone. Have a system in place to review the relevance of the metrics frequently. Metrics should definitely be reviewed at the end of each development cycle but can also be an item for discussion during monthly meetings to ensure sufficient flexibility without being too burdensome. During the review, again focus on both the what and the how. Many teams concentrate on what metrics to measure and get frustrated with unreliable measurements as the exact mechanisms of measurement are poorly defined.
Having the right system in place is already 90% of the work done. The last bit has to do with actually defining the metrics and method of measurements. Below are three tips for creating and choosing metrics:
- Source for similar metrics from the market: I mentioned earlier that there is a dearth of applicable metrics available in the market for novel deep tech products. While many deep tech technologies are ground-breaking, the market pain points they are relieving are already in existence. Take EV for example, while the technology itself might have been innovative back when it started, the market needs for transportation from point A to point B is already well-established. Thus, product development teams should always identify the market that the product would potentially cater towards, and pinpoint critical metrics already in used by the market. Some of the metrics identified are immediately translatable to the new product but most would not be. The idea here is to take what is relevant, tweak what is possible and discard the rest. Many internal and external stakeholders would attempt to benchmark the new product against market alternatives with well-established metrics so be clear minded about the similarities and differences between internally adopted metrics and existing metrics available in the market.
- Application driven: When formulating metrics, product development teams can also derive inspirations from potential applications of the product. For instance, towing capacity might be an irrelevant metric for regular family sedan but highly necessary for tow trucks. Once the target product application is defined, teams should review the metrics to ensure comprehensiveness of the list. Discard keep application-driven metrics for applications that are actively being developed to reduce data redundancies and data collection overhead.
Created from scratch: Even after scouring and tweaking everything in the market, teams might realise that the available metrics are still inadequate for capturing the performance of the product. Creating metrics from scratch is a fun process but it requires persistence. New metrics are hardly created right the first-time round. The mechanism of measurement is especially important for new metrics. Once a new metric is created, keep a tight lookout for the limitations and failures of the metric so timely revisions can be implemented. New metrics are typically met with distrusts and criticisms both internally and externally which should be seen as a feedback process.
6. Value uncertainties
Novel products generally face problems with value creation. Because the product is so new, customers and the market do not thoroughly grasp its value and potential use-case. If customers have to perform a lot of mental gymnastics to understand the product, they will need to put in even more effort to apply the product and generate value out of it. That is a tall order for more people. Products that are more similar to alternatives in the market can penetrate the market more readily than novel products can. Admittedly, this is true for any technology, not just deep tech. However, value creation for deep tech products is more challenging because the time to build up complementary products in the projected value chain is much higher.

Series value chain. Value is created as it moves along the value chain
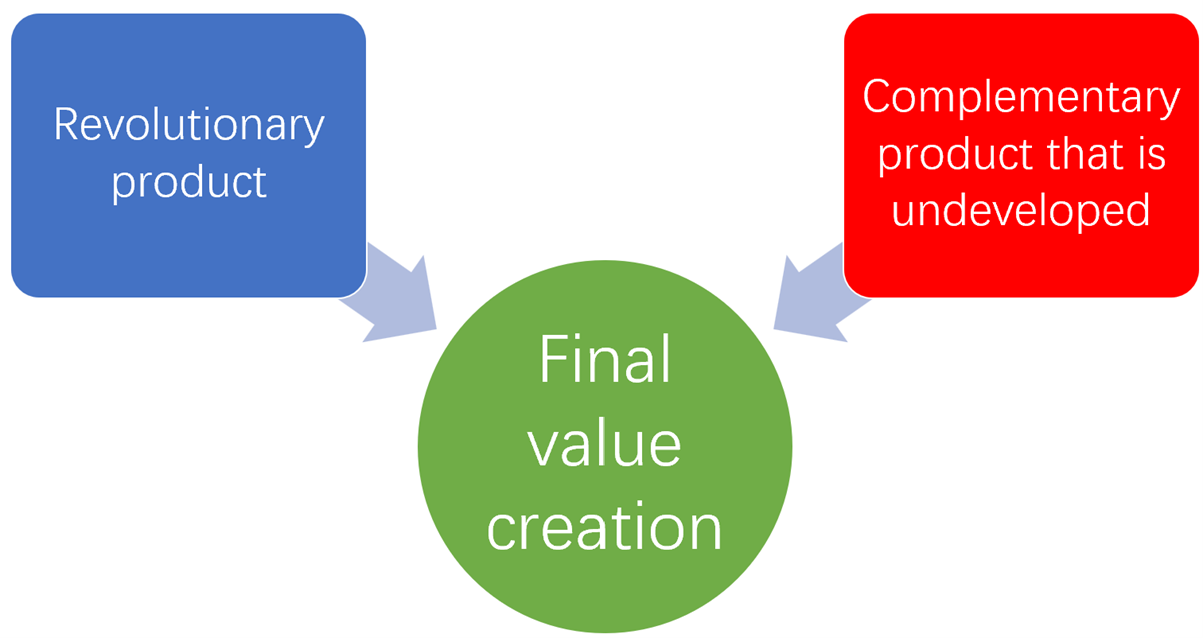
Parallel value chain. Complementary products come together to create final value
Value can be created either in series or in parallel. Products can only exist in markets with either series, parallel or mixed value chains. There are some deep tech products that can deliver value by itself or in conjunction with other existing products. However, some deep tech products need to be supported by or fed into multiple other hitherto non-existent products before value can be generated. This is frustrating because for deep tech, many of the non-existent products are also deep tech and thus the development burden becomes insurmountable. In addition, many deep tech are more complicated and niche in nature rending it difficult to find partners willing to assist with complementary product development.
For deep tech, managing value uncertainties hinges on two things:
- Digestible product: Make the product as easily understandable as possible. While the technology itself might be too complicated to be simplified, the operation, inputs and outputs of the product should be straightforward. Avoid products that can be customized a thousand different ways for as many applications during the initial release of the product. Having a two/three liner description about the what and the how of the product is helpful. The less energy the customer spends on deciphering the product, the more he or she can imagine use-cases for the product. In trying to make a product comprehensible, it is sometimes necessary to apply the product on a use-case which is familiar to the market. This requires additional product development work. I call this seeding, as it allows the market to warm up to the idea of using the product for the validated use-cases, which would eventually invite greater room for imagination. For instance, high throughput oligopool is a promising product but the market might be unsure about its performance in actual applications. Internally driven validation runs accompanied by corresponding data on how the oligopool product can be used for target enrichment allows the market to be more confident with the capabilities of oligopool.
- Ecosystem construction: Work on developing the value chain as it indirectly affects the value of the product. Ecosystem construction is something that should be strategically considered early during development as it takes time for different players and products involved in the ecosystem to mature. The first step is to be keenly aware of the type of value chains that exist in the ecosystem. Products in parallel value chain can usually realize some value by themselves but perform much better when paired with complementary products. For example, if we bring an EV back in time 30 years when public charging stations are unavailable, the EV can already provide limited value to the owner (assuming there is a way to charge the car at home). Having abundant public charging stations elevates the value of the EV to a much higher level. Products in series value chain are only of value to the next step in the value chain. For example, if we bring an AI chip back 30 years, it would be a useless product by itself as AI computation was not developed. Value creation for series value chain is most difficult as it typically runs into the chicken and egg problem. If AI computation is non-existent, AI chip would not be developed as it is of little value and vice-versa. Once the value ecosystem is clearly defined, identify weakness in the value chain and look for ways to rectify the situation. Methods can include partnerships, alternatives sourcing or internal development. Start working on strengthening the value chain early to avoid being caught in a situation where the core product is ready but the value chain is broken.
I hope the information and tips provided in this article is helpful for your product development. Feel free to leave a comment.
Add comment
Comments